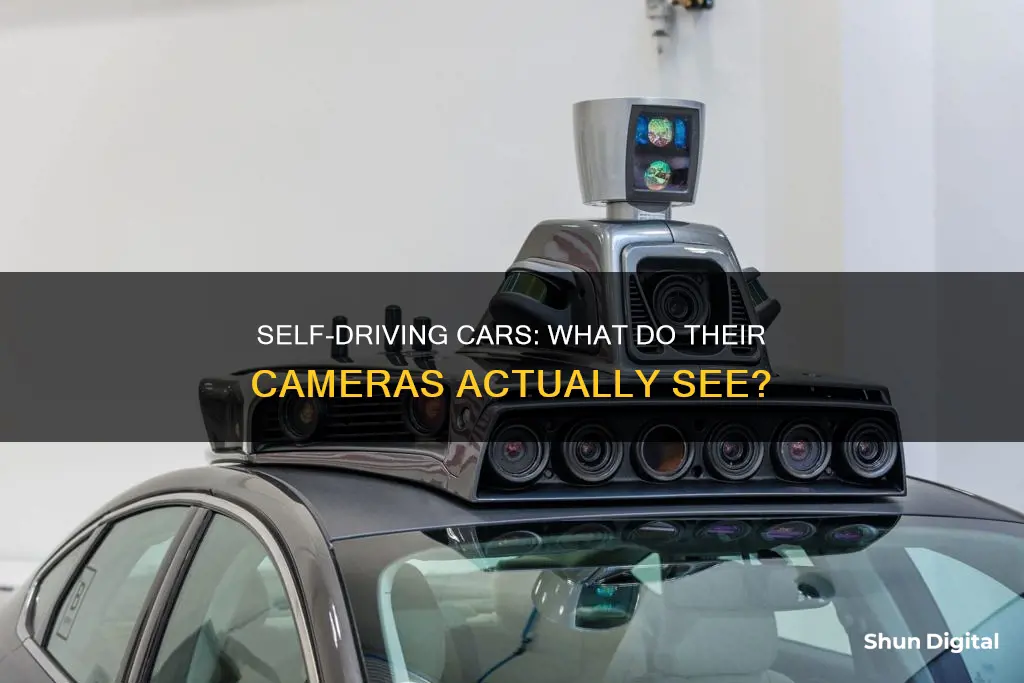
Self-driving cars use a combination of cameras, radar, and lidar to see their surroundings. Cameras are placed on every side of the car to provide a 360-degree view of the environment. They have a wide field of view, ranging from wide-angle lenses that capture close-up surroundings to longer, narrower lenses that focus on what's ahead. While cameras offer the highest-resolution images, they struggle in low-visibility conditions like fog, rain, or at night. To overcome this, self-driving cars also use radar and lidar sensors, which emit radio waves or laser light pulses that bounce off objects and return to the sensor, providing data on the speed, location, and shape of surrounding objects.
Characteristics | Values |
---|---|
Camera placement | Front, rear, left, and right |
Field of view | Up to 120 degrees |
Camera type | Fish-eye, wide-angle, or narrow-angle |
Camera function | Stitching together a 360-degree view, providing visuals of surroundings, lane lines, and road signs |
Limitations | Difficulty in detecting objects in low-visibility conditions and calculating the distance of objects |
Radar function | Detecting speed, location, and nearby objects; supplementing camera vision in low-visibility conditions |
Lidar function | Creating a 3D view of the environment, including the shape and depth of objects and road geography; detecting velocity |
What You'll Learn
Limitations of cameras in self-driving cars
Cameras are one of the most popular means of environmental sensing for self-driving cars, as they visually sense the environment in the same way humans do. However, there are several limitations to using cameras in self-driving cars.
Firstly, cameras do not work well in all weather conditions. They are affected by lighting conditions, such as strong shadows, bright lights, or low-light situations, which can cause confusion and impact the camera's ability to detect objects accurately. For example, in a test by Luminar, a Tesla Model Y was unable to detect and avoid a dummy pedestrian child in low-light conditions.
Secondly, cameras require more computational power than other sensing technologies. Camera-based systems must ingest and analyze images to determine the distance and speed of objects, whereas technologies like LiDAR (Light Detection and Ranging) can immediately tell the distance and direction of an object. This additional processing requires more powerful onboard computers, which can increase costs.
Thirdly, cameras are limited in their range-detecting capabilities. While they can provide a 360-degree view of the surrounding environment, they do not have the same range as LiDAR or radar systems. This limitation can impact the ability of self-driving cars to detect objects at longer distances and make necessary adjustments.
Additionally, critics argue that cameras still cannot see well enough to avoid danger, especially in adverse weather conditions. They need to accurately perceive the environment in all types of conditions, just as a human driver would.
Furthermore, cameras are "dumb" sensors in that they only provide raw image data. Camera systems rely on powerful machine learning and neural networks to process those images and extract meaningful information, such as object detection and distance estimation. This requires significant computational resources and advanced algorithms, adding complexity to the system.
Lastly, cameras may face challenges with interference and jamming when multiple vehicles with camera systems are operating in close proximity. The exact impact of this potential issue is not yet fully understood and requires further investigation.
While cameras offer high-resolution images and are essential for certain tasks like reading road signs, they have limitations when used as the sole sensing technology in self-driving cars. Combining cameras with other sensors, such as LiDAR or radar, can help mitigate these limitations and improve the overall safety and performance of self-driving vehicles.
USB Camera Setup: Windows 10 Mini USB Spy Camera Guide
You may want to see also
How cameras help self-driving cars see
Cameras are a critical component of self-driving cars. They are placed on every side of the vehicle to stitch together a 360-degree view of its environment. Some have a wide field of view, while others focus on a more narrow view to provide long-range visuals. The cameras used in autonomous cars are specialized image sensors that detect the visible light spectrum reflected from objects.
Cameras are the most accurate way to create a visual representation of the world, especially when it comes to self-driving cars. They offer the highest-resolution images and are the best way to get an accurate view of a vehicle's surroundings. This makes them incredibly useful for reading road signs and markings, and for understanding the vehicle's relative position within its environment.
However, cameras have limitations. They struggle in low-visibility conditions, such as fog, rain, or at night. They also require the computer to take measurements from an image to understand how far away an object is, unlike radar and lidar, which provide numerical data.
To overcome these limitations, self-driving cars combine camera data with other sensors, such as radar and lidar. Radar sensors, which work by transmitting radio waves, can supplement camera vision in low-visibility conditions and improve object detection. On the other hand, lidar uses laser light pulses to create a 3D model of the car's surroundings, providing shape and depth to surrounding objects and the road.
By fusing data from multiple sensors, self-driving cars can "see" their environment better than human eyesight and make more informed driving decisions. This combination of sensors ensures reliability and redundancy, allowing the vehicle to verify the accuracy of its detections and eliminate blind spots.
In conclusion, cameras play a crucial role in helping self-driving cars see and understand their surroundings. When combined with other sensors, they enable autonomous vehicles to perceive and interpret their environment, make driving decisions, and enhance safety on the road.
Canada's Camera TV Show: How Long Did It Last?
You may want to see also
Cameras vs radar and lidar sensors
Self-driving cars use several technologies to create their maps of the world, including cameras, radar, and lidar.
Cameras are the most accurate way to create a visual representation of the world. They can be placed on every side of a car to stitch together a 360-degree view of its environment. Some have a wide field of view, while others focus on a more narrow view to provide long-range visuals. Fish-eye cameras, for example, contain super-wide lenses that provide a panoramic view, which is useful for parking.
However, cameras have their limitations. They struggle in low-visibility conditions, such as fog, rain, or at night. They can distinguish details of the surrounding environment, but the distances of those objects need to be calculated.
Radar sensors use radio waves for object recognition and detection. They can detect objects and measure their distance and speed in real time. Both long-range and short-range radar sensors are available, each serving a different purpose. Short-range radar is used for tasks like monitoring blind spots, lane-assist, and parking aids, while long-range radar helps cars keep a safe distance from other vehicles and offers brake assistance. Radar works just as well in poor weather conditions, but it is only 90-95% effective at detecting pedestrians, and most sensors are 2-dimensional, making it impossible to accurately determine the height of an object.
Lidar sensors are similar to radar but use laser beams instead of radio waves. They provide accurate distance measurements and create a 3D point cloud that maps a 360-degree view around the car. Lidar works in just about every lighting condition and is highly reliable, but it is expensive and requires more space to implement on cars.
To be fully autonomous, cars will likely require a combination of camera, radar, and lidar sensors, as each technology has its own advantages and disadvantages.
Exploring F1 Onboard Cameras: A Spectator's Guide
You may want to see also
How cameras create a visual representation of the world
Cameras are one of the three primary sensors used in self-driving cars to create a visual representation of the world. Autonomous vehicles rely on cameras placed on every side—front, rear, left, and right—to stitch together a 360-degree view of their environment. Some have a wide field of view of up to 120 degrees and a shorter range, while others focus on a narrower view to provide long-range visuals. Fish-eye cameras with super-wide lenses are also used to give a full picture of what's behind the vehicle for parking assistance.
These cameras provide accurate visuals and can distinguish details of the surrounding environment, but they have limitations in calculating the exact distance of objects. They also face challenges in low-visibility conditions, such as fog, rain, or at night. To overcome these limitations, cameras are often used in conjunction with other sensors like radar and lidar, which provide complementary data on speed, location, and the three-dimensional shape of objects.
The data from these multiple sensors is then fused using a process called sensor fusion, where relevant portions of data are combined by a centralized AI computer to make driving decisions. This ensures reliability and redundancy, allowing the autonomous vehicle to create a comprehensive and up-to-date visual representation of its surroundings.
Are Gas Stations Closely Monitoring You?
You may want to see also
The future of cameras in self-driving cars
The Case for Camera-Only Systems
The argument for camera-only self-driving cars is based on the idea that humans drive using only their vision, so a machine should be able to do the same. This approach relies on the continued development of artificial intelligence (AI) and deep learning to process visual data as humans do. Tesla is a prominent advocate for this method and has invested heavily in neural networks and camera input to estimate depth, velocity, and acceleration. They have also developed an auto-labelling system that speeds up the process of annotating training examples, allowing them to scale their efforts efficiently.
The Multi-Sensor Approach
On the other hand, many self-driving car companies are reluctant to rely solely on image processing and continue to favour the use of LiDAR and other sensory data. This is because cameras have limitations, such as difficulty in distinguishing the distance of objects and performing poorly in low-visibility conditions like fog, rain, or nighttime driving. To overcome these challenges, a multi-sensor approach combines cameras with other technologies like LiDAR and radar. LiDAR uses light detection and ranging to create a 3D map of the surroundings, while radar detects the speed and location of objects. Together, these sensors provide complementary data to improve safety and performance in various conditions.
Future Developments
While the debate continues, advancements in camera technology and AI are pushing the boundaries of what is possible. New stereo cameras, which use two perspectives to establish depth, are poised to revolutionize autonomous car safety. These short- and long-range cameras generate stereoscopic 3D point clouds, achieving 'pseudo-LiDAR' visualization of surroundings. Additionally, machine learning enables 3D-equipped cars to resolve objects better and react more quickly than human drivers. As research progresses, it is likely that we will see a combination of camera-only and multi-sensor approaches, tailored to specific use cases and conditions.
Running Q-See Camera Cables: Through-Wall Installation Guide
You may want to see also
Frequently asked questions
Self-driving cars use a combination of cameras, radar, and lidar sensors to see their surroundings.
Cameras are placed on every side of self-driving cars to stitch together a 360-degree view of their environment. Some have a wide field of view and a shorter range, while others focus on a narrower view to provide long-range visuals.
Cameras have difficulty detecting objects in low-visibility conditions, such as fog, rain, or at night. They can distinguish details of the surrounding environment, but the distances of those objects need to be calculated to know their exact location.
By combining different types of sensors, self-driving cars can create a detection system that "sees" a vehicle's environment even better than human eyesight. This system provides diversity and redundancy, with overlapping sensors verifying the accuracy of the data collected.